Investigating the Relationship Between Resting-state EEG Frontoparietal Coherence, Visuospatial Ability, and Motor Skill Acquisition: A Retrospective Analysis
DOI:
https://doi.org/10.15540/nr.9.2.82Keywords:
visuospatial function, EEG, imaginary coherence, motor learningAbstract
Introduction: Visuospatial ability may explain individual differences in the extent of motor skill learning. This study tested whether frontoparietal functional connectivity at rest, measured by resting-state electroencephalography (EEG) coherence, is related to both visuospatial performance and motor skill acquisition (an early stage of motor learning). Methods: Across 21 participants, the following data were retrospectively analyzed: 2-min eyes-closed resting-state EEG, the Visuospatial/Constructional Index score from the Repeatable Battery for the Assessment of Neuropsychological Status (RBANS), and five practice trials of a functional motor task. Right frontoparietal coherence in the alpha band (8–12 Hz) was computed with imaginary coherence (IC) between electrodes F4 and P4, with ICs from left and midline electrodes included as negative controls. Results: F4–P4 alpha IC was highly correlated with the RBANS Visuospatial/Constructional Index, while left and midline alpha ICs were not. However, there was no correlation between right frontoparietal alpha IC with skill acquisition. Conclusion: This study supports that right frontoparietal IC is positively related with visuospatial function, yet the limited dose of motor practice (five trials) in the retrospective dataset was not inherently designed to investigate motor skill acquisition per se. However, results show proof of concept for developing right frontoparietal alpha IC-based neurofeedback applications for visuospatial training.
References
Brandes-Aitken, A., Anguera, J. A., Chang, Y.-S., Demopoulos, C., Owen, J. P., Gazzaley, A., Mukherjee, P., & Marco, E. J. (2019). White matter microstructure associations of cognitive and visuomotor control in children: A sensory processing perspective. Frontiers in Integrative Neuroscience, 12, 65. https://doi.org/10.3389/fnint.2018.00065
Brooks, V., Hilperath, F., Brooks, M., Ross, H. G., & Freund, H. J. (1995). Learning “what” and “how” in a human motor task. Learning & Memory, 2(5), 225–242. https://doi.org/10.1101/lm.2.5.225
Caselli, R. J., Langlais, B. T., Dueck, A. C., Chen, Y., Su, Y., Locke, D. E. C., Woodruff, B. K., & Reiman, E. M. (2020). Neuropsychological decline up to 20 years before incident mild cognitive impairment. Alzheimer’s & Dementia, 16(3), 512–523. https://doi.org/10.1016/j.jalz.2019.09.085
Cohen, M. X. (2015). Comparison of different spatial transformations applied to EEG data: A case study of error processing. International Journal of Psychophysiology, 97(3), 245–257. https://doi.org/10.7551/mitpress/9609.001.0001
Cohen, M. X. (2014). Analyzing neural time series data: Theory and practice. Cambridge, MA: The MIT Press. https://doi.org/10.1017/CBO9781107415324.004
Corbetta, M., Kincade, J. M., Ollinger, J. M., McAvoy, M. P., & Shulman, G. L. (2000). Voluntary orienting is dissociated from target detection in human posterior parietal cortex. Nature Neuroscience, 3, 292–297. https://doi.org/10.1038/73009
de Cheveigné, A. (2020). ZapLine: A simple and effective method to remove power line artifacts. NeuroImage, 207, 116356. https://doi.org/10.1016/j.neuroimage.2019.116356
Delorme, A., & Makeig, S. (2004). EEGLAB: An open source toolbox for analysis of single-trial EEG dynamics including independent component analysis. Journal of Neuroscience Methods, 134(1), 9–21. https://doi.org/10.1016/j.jneumeth.2003.10.009
Delorme, A., Sejnowski, T., & Makeig, S. (2007). Enhanced detection of artifacts in EEG data using higher-order statistics and independent component analysis. NeuroImage, 34(4), 1443–1449. https://doi.org/10.1016/j.neuroimage.2006.11.004
Foxe, J. J., McCourt, M. E., & Javitt, D. C. (2003). Right hemisphere control of visuospatial attention: Line-bisection judgments evaluated with high-density electrical mapping and source analysis. NeuroImage, 19(3), 710–726. https://doi.org/10.1016/S1053-8119(03)00057-0
Hari, R., & Salmelin, R. (1997). Human cortical oscillations: A neuromagnetic view through the skull. Trends in Neurosciences, 20(1), 44–49. https://doi.org/10.1016/S0166-2236(96)10065-5
Hillebrand, A., Barnes, G. R., Bosboom, J. L., Berendse, H. W., & Stam, C. J. (2012). Frequency-dependent functional connectivity within resting-state networks: An atlas-based MEG beamformer solution. NeuroImage, 59(4), 3909–3921. https://doi.org/10.1016/j.neuroimage.2011.11.005
Hoffmann, S., & Falkenstein, M. (2008). The correction of eye blink artefacts in the EEG: A comparison of two prominent methods. PLoS ONE, 3(8), e3004. https://doi.org/10.1371/journal.pone.0003004
Jensen, O., Goel, P., Kopell, N., Pohja, M., Hari, R., & Ermentrout, B. (2005). On the human sensorimotor-cortex beta rhythm: Sources and modeling. NeuroImage, 26(2), 347–355. https://doi.org/10.1016/j.neuroimage.2005.02.008
Johnson, D. K., Storandt, M., Morris, J. C., & Galvin, J. E. (2009). Longitudinal study of the transition from healthy aging to Alzheimer disease. Archives of Neurology, 66(10), 1254–1259. https://doi.org/10.1001/archneurol.2009.158
Jokinen, H., Melkas, S., Ylikoski, R., Pohjasvaara, T., Kaste, M., Erkinjuntti, T., & Hietanen, M. (2015). Post-stroke cognitive impairment is common even after successful clinical recovery. European Journal of Neurology, 22(9), 1288–1294. https://doi.org/10.1111/ene.12743
Jongbloed, L. (1986). Prediction of function after stroke: A critical review. Stroke, 17(4), 765–776. https://doi.org/10.1161/01.str.17.4.765
Kayser, J., & Tenke, C. E. (2015). Issues and considerations for using the scalp surface Laplacian in EEG/ERP research: A tutorial review. International Journal of Psychophysiology, 97(3), 189–209. https://doi.org/10.1016/j.ijpsycho.2015.04.012
Keil, A., Debener, S., Gratton, G., Junghöfer, M., Kappenman, E. S., Luck, S. J., Miller, G. A., & Yee, C. M. (2014). Committee report: Publication guidelines and recommendations for studies using electroencephalography and magnetoencephalography. Psychophysiology, 51(1), 1–21. https://doi.org/10.1111/psyp.12147
Kumar, A., Panthi, G., Divakar, R., & Mutha, P. K. (2020). Mechanistic determinants of effector-independent motor memory encoding. Proceedings of the National Academy of Sciences of the United States of America, 117(29), 17338–17347. https://doi.org/10.1073/pnas.2001179117
Lingo VanGilder, J., Hengge, C. R., Duff, K., & Schaefer, S. Y. (2018). Visuospatial function predicts one-week motor skill retention in cognitively intact older adults. Neuroscience Letters, 664, 139–143. https://doi.org/10.1016/j.neulet.2017.11.032
Lingo VanGilder, J., Lohse, K. R., Duff, K., Wang, P., & Schaefer, S. Y. (2021). Evidence for associations between Rey-Osterrieth Complex Figure test and motor skill learning in older adults. Acta Psychologica, 214, 103261. https://doi.org/10.1016/j.actpsy.2021.103261
Manuel, A. L., Guggisberg, A. G., Thézé, R., Turri, F., & Schnider, A. (2018). Resting-state connectivity predicts visuo-motor skill learning. NeuroImage, 176, 446–453. https://doi.org/10.1016/j.neuroimage.2018.05.003
Mottaz, A., Corbet, T., Doganci, N., Magnin, C., Nicolo, P., Schnider, A., & Guggisberg, A. G. (2018). Modulating functional connectivity after stroke with neurofeedback: Effect on motor deficits in a controlled cross-over study. NeuroImage: Clinical, 20, 336–346. https://doi.org/10.1016/j.nicl.2018.07.029
Mottaz, A., Solcà, M., Magnin, C., Corbet, T., Schnider, A., & Guggisberg, A. G. (2015). Neurofeedback training of alpha-band coherence enhances motor performance. Clinical Neurophysiology, 126(9), 1754–1760. https://doi.org/10.1016/j.clinph.2014.11.023
Nolte, G., Bai, O., Wheaton, L., Mari, Z., Vorbach, S., & Hallett, M. (2004). Identifying true brain interaction from EEG data using the imaginary part of coherency. Clinical Neurophysiology, 115(10), 2292–2307. https://doi.org/10.1016/j.clinph.2004.04.029
Nunez, P. L., & Srinivasan, R. (2009). Electric Fields of the Brain: The neurophysics of EEG. Oxford Scholarship Online. https://doi.org/10.1093/acprof:oso/9780195050387.001.0001
Oostenveld, R., & Praamstra, P. (2001). The five percent electrode system for high-resolution EEG and ERP measurements. Clinical Neurophysiology, 112(4), 713–719. https://doi.org/10.1016/s1388-2457(00)00527-7
Pathania, A., Euler, M. J., Clark, M., Cowan, R. L., Duff, K., & Lohse, K. R. (2022). Resting EEG spectral slopes are associated with age-related differences in information processing speed. Biological Psychology, 168, 108261. https://doi.org/10.1016/j.biopsycho.2022.108261
Perrin, F., Pernier, J., Bertrand, O., & Echallier, J. F. (1989). Spherical splines for scalp potential and current density mapping. Electroencephalography and Clinical Neurophysiology, 72(2), 184–187. https://doi.org/10.1016/0013-4694(89)90180-6
Pion-Tonachini, L., Kreutz-Delgado, K., & Makeig, S. (2019). ICLabel: An automated electroencephalographic independent component classifier, dataset, and website. NeuroImage, 198, 181–197. https://doi.org/10.1016/j.neuroimage.2019.05.026
Plöchl, M., Ossandón, J., & König, P. (2012). Combining EEG and eye tracking: Identification, characterization, and correction of eye movement artifacts in electroencephalographic data. Frontiers in Human Neuroscience, 6, 278. https://doi.org/10.3389/fnhum.2012.00278
Randolph, C., Tierney, M. C., Mohr, E., & Chase, T. N. (1998). The repeatable battery for the assessment of neuropsychological status (RBANS): Preliminary clinical validity. Journal of Clinical and Experimental Neuropsychology, 20(3), 310–319. https://doi.org/10.1076/jcen.20.3.310.823
Regan, E., Fridriksson, J., Schaefer, S. Y., Rorden, C., Bonilha, L., Lingo VanGilder, J., & Stewart, J. C. (2021). Neural correlates of within-session practice effects in mild motor impairment after stroke: A preliminary investigation. Experimental Brain Research, 239(1), 151–160. https://doi.org/10.1007/s00221-020-05964-y
Rizk, S., Ptak, R., Nyffeler, T., Schnider, A., & Guggisberg, A. G. (2013). Network mechanisms of responsiveness to continuous theta-burst stimulation. European Journal of Neuroscience, 38(8), 3230–3238. https://doi.org/10.1111/ejn.12334
Schaefer, S. Y., & Duff, K. (2017). Within-session and one-week practice effects on a motor task in amnestic mild cognitive impairment. Journal of Clinical and Experimental Neuropsychology, 39(5), 473–484. https://doi.org/10.1080/13803395.2016.1236905
Sitaram, R., Ros, T., Stoeckel, L., Haller, S., Scharnowski, F., Lewis-Peacock, J., Weiskopf, N., Blefari, M. L., Rana, M., Oblak, E., Birbaumer, N., & Sulzer, J. (2017). Closed-loop brain training: The science of neurofeedback. Nature Reviews Neuroscience, 18(2), 86–100. https://doi.org/10.1038/nrn.2016.164
Steele, C. J., Scholz, J., Douaud, G., Johansen-Berg, H., & Penhune, V. B. (2012). Structural correlates of skilled performance on a motor sequence task. Frontiers in Human Neuroscience, 6, 289. https://doi.org/10.3389/fnhum.2012.00289
Tenke, C. E., & Kayser, J. (2012). Generator localization by current source density (CSD): Implications of volume conduction and field closure at intracranial and scalp resolutions. Clinical Neurophysiology, 123(12), 2328–2345. https://doi.org/10.1016/j.clinph.2012.06.005
Wang, P., Infurna, F. J., & Schaefer, S. Y. (2020). Predicting motor skill learning in older adults using visuospatial performance. Journal of Motor Learning and Development, 8(1), 38–51. https://doi.org/10.1123/jmld.2018-0017
Wu, J., Knapp, F., Cramer, S. C., & Srinivasan, R. (2018). Electroencephalographic connectivity measures predict learning of a motor sequencing task. Journal of Neurophysiology, 119(2), 490–498. https://doi.org/10.1152/jn.00580.2017
Wu, J., Srinivasan, R., Kaur, A., & Cramer, S. C. (2014). Resting-state cortical connectivity predicts motor skill acquisition. NeuroImage, 91, 84–90. https://doi.org/10.1016/j.neuroimage.2014.01.026
Zhou, R. J., Hondori, H. M., Khademi, M., Cassidy, J. M., Wu, K. M., Yang, D. Z., Kathuria, N., Erani, F. R., Dodakian, L., McKenzie, A., Lopes, C. V., Scacchi, W., Srinivasan, R., & Cramer, S. C. (2018). Predicting gains with visuospatial training after stroke using an EEG measure of frontoparietal circuit function. Frontiers in Neurology, 9, 597. https://doi.org/10.3389/fneur.2018.00597
Zoefel, B., Huster, R. J., & Herrmann, C. S. (2011). Neurofeedback training of the upper alpha frequency band in EEG improves cognitive performance. NeuroImage, 54(2), 1427–1431. https://doi.org/10.1016/j.neuroimage.2010.08.078
Downloads
Published
Issue
Section
License
Authors who publish with this journal agree to the following terms:- Authors retain copyright and grant the journal right of first publication with the work simultaneously licensed under a Creative Commons Attribution License (CC-BY) that allows others to share the work with an acknowledgement of the work's authorship and initial publication in this journal.
- Authors are able to enter into separate, additional contractual arrangements for the non-exclusive distribution of the journal's published version of the work (e.g., post it to an institutional repository or publish it in a book), with an acknowledgement of its initial publication in this journal.
- Authors are permitted and encouraged to post their work online (e.g., in institutional repositories or on their website) prior to and during the submission process, as it can lead to productive exchanges, as well as earlier and greater citation of published work (See The Effect of Open Access).
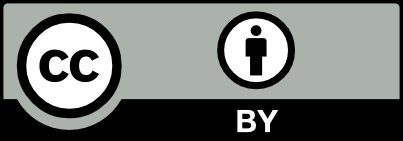